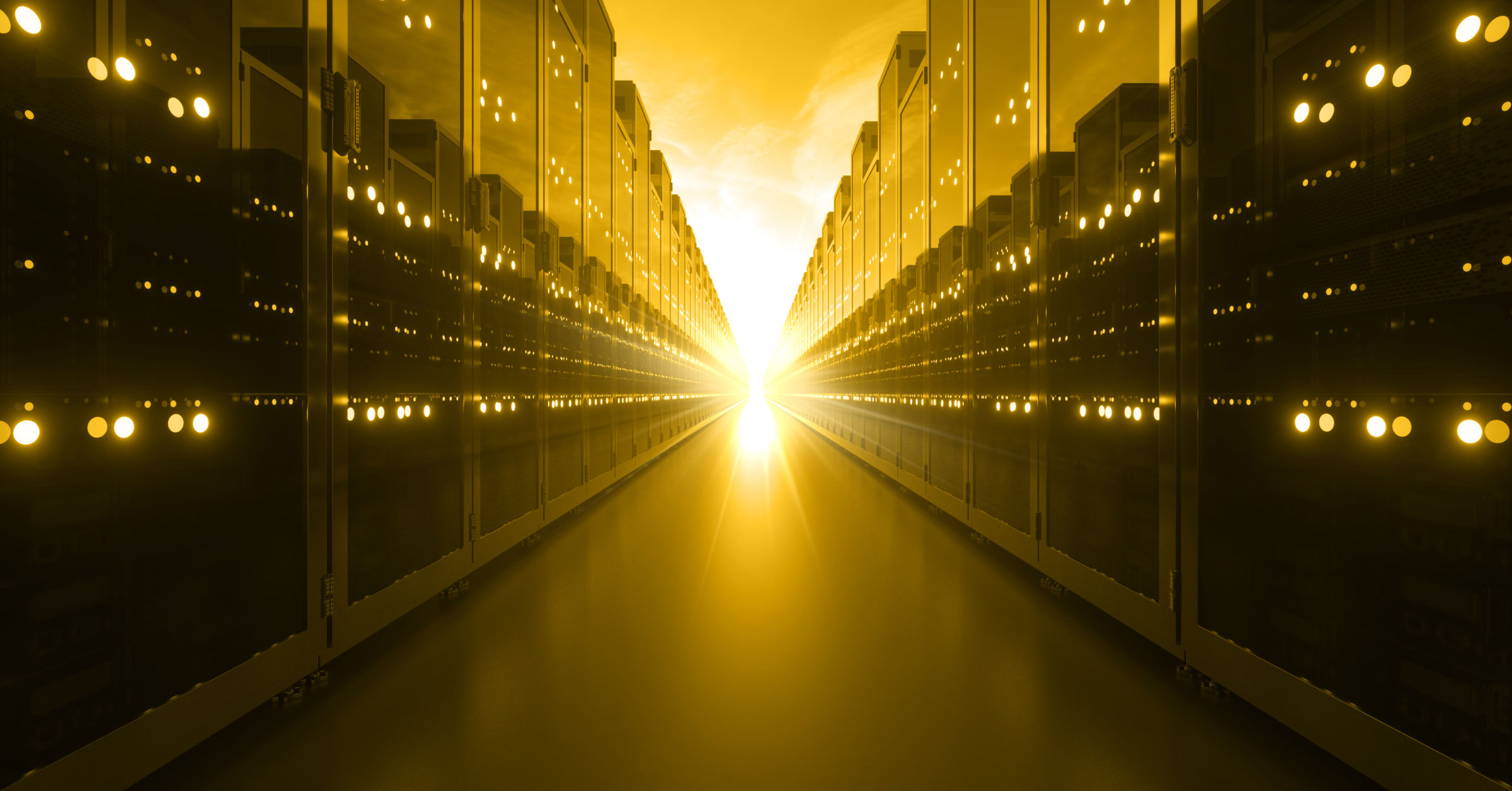
A Brief History
I was thinking recently about the evolution of digital security over the past 25 years. We went from a world of wired connections and office buildings to global networks of cloud resources that are accessed as often from phones and tablets as they are from corporately managed devices. We lived in a world where security consisted of a firewall, antivirus, and a keycard to access the building. And now the average enterprise organization has more than twenty-five security tools in their stack. We came from a world where “the IT guy” oversaw security to one where we have red teams, blue teams, purple teams, SOC analysts, incident responders, threat hunters, and more. Attacks used to cause a user’s PC to pop-up ads for sketchy websites. Now they encrypt an organization’s data, compromise SSO credentials and exfiltrate critical intellectual property for sale to the highest bidder. To say that the increase in complexity has been exponential would be a glaring understatement. And yet, one thing has remained constant in this world of upheaval. The protection of organizations’ digital assets still falls to human beings. And the front line of defense is the SOC analyst.
The Challenge
Unfortunately, this evolution of digital threats did not come with a corresponding evolution in the ability to detect and respond to them. The evolution in tools, although necessary, also created its own problems. Every tool operates in its own silo, and generates alerts based on its own narrow view of the world. With over twenty tools, each generating alerts, the volume of data being thrown at the SOC analyst quickly became overwhelming (and is getting worse).
The Pain
What was the result of this explosion of tools?
Security:
- Missed Detections: The increased number of alerts and the high volume of noise (i.e. false positives) leads to real alerts being dismissed due to Alert Fatigue.
- Inadequate Response: Even when an event is properly detected, it requires time and analyst expertise to understand the breadth of the incident. Increasingly complex attacks may not be fully understood and only partially remediated, leaving an attacker access to the network.
Organization:
- Staffing: Too many alerts, not enough time, and the stress of the outcome for missed detections leads to analyst burnout. Mated to the tedious nature of triaging thousands of (mostly false) alerts, with little meaningful proactive work, low job satisfaction and turnover often follow.
- Cost: The human cost of staffing a SOC has skyrocketed. Experienced security practitioners who can understand the data generated by dozens of disparate tools, and correlate this data, are hard to find. This, coupled with the volume of people required to triage hundreds or thousands of alerts per day, creates a need for a large, skilled security operations team.
The Solution! (Or was it?)
In 2005, Gartner coined the term “SIEM” in a report called “Improve IT Security with Vulnerability Management.” This ushered in a new era (and a new Magic Quadrant of vendors) to address the pains caused by the explosion of siloed tools. The promise was a simple one: Get insight into the security of the organization by centralizing the collection of this siloed data and tying together all of the “loose threads” into cohesive stories. But the devil is always in the details.
I remember one of the first lessons I learned in my freshman class “Intro to Programming Logic” was “Garbage In, Garbage Out”. And this principle became increasingly noticeable with the rapid adoption of the SIEM. Ever-increasing volumes of data didn’t provide clarity or insight, they provided higher volumes of noise. Organizations dedicated significant resources to fixing this problem, and it became a never-ending battle of “tuning the SIEM” (i.e. manually tweaking the data, the queries, the rules and alerts to minimize the amount of unimportant, or outright inaccurate data coming out of the platform). The SIEM was only as valuable as the expertise of the people who managed it (and finding these people was a significant challenge). The problem became so pronounced that a survey of security leaders by 451 Research revealed that only 21.6% of organizations felt that they were getting the value out of the SIEM that they were expecting.
But the Times, They Are A-Changin’
Although SIEM had struggled to deliver on its full potential, the core concept was a good one. All it needed was a nudge from another field of computing. And it got one in the form of AI. Although technically around since the 1950s, AI has experienced a golden age in the last 10 years due to the advances in Deep Learning, Big Data, and Large Language Models. Although Chat GPT (and its ilk) get all the publicity for the generative AI cat memes, it is the Deep Learning and Big Data modeling that have the biggest impact for security teams. These technologies enabled a massive leap forward in Narrow (or “Weak”) AI. At its core, these systems can analyze large datasets more quickly and accurately than humans, identify patterns and trends, and make data-driven predictions or decisions. They are also capable of learning and improving over time through techniques such as machine learning, particularly deep learning, where they can adjust their algorithms based on the data they process.
Sounds Cool but How Does This Solve “The SIEM Problem”?
As mentioned earlier, SIEM’s goal was to provide global insight based on the correlation of vast amounts of data. But unfortunately, it fell to human beings to make this happen, and quite frankly, we did it poorly. Narrow AI or Artificial Narrow Intelligence (ANI) was purpose built for this type of work. It is an Expert System that can simultaneously analyze tens of thousands of unique pieces of data, correlate them into a single meaningful chain of events, and discern not only the connections between them, but the bigger meaning of the incident.
Can I Fire My SOC Team Then?
Well let’s not get ahead of ourselves…
What ANI can provide, in conjunction with a large, centralized data store, is the insight that used to only be achieved through many hours of tedious log searches, and manual event correlation. If implemented well, Narrow AI can eliminate the need for alert triage completely. Well trained models will evaluate every alert and do a continuous assessment of each threat in the greater context of environment. SOC analysts only need to get involved when there is enough evidence to justify that an alert (or series of alerts) is a legitimate security incident. And when they do, they should have all the relevant data provided so that no remnant of the attack remains after remediation.
So instead of going through the 500th alert of the day your team can be threat hunting through all that collected data, or researching the latest MITRE TTPs, or working on the next security cert. But whatever they choose to do with this time, it will be more rewarding, while still providing top notch security operations. And who doesn’t want that?
Wrapping it Up
It’s been a crazy quarter of a century and I’m sure this is just the beginning. But I am confident that we can meet the challenges of the latest cyber threats and APT assaults if we learn to leverage the tools at our disposal. Personally, I wholeheartedly welcome the wonderful new world of AI. (But I reserve the right to change my mind when SKYNET becomes self aware).